Table Of Content
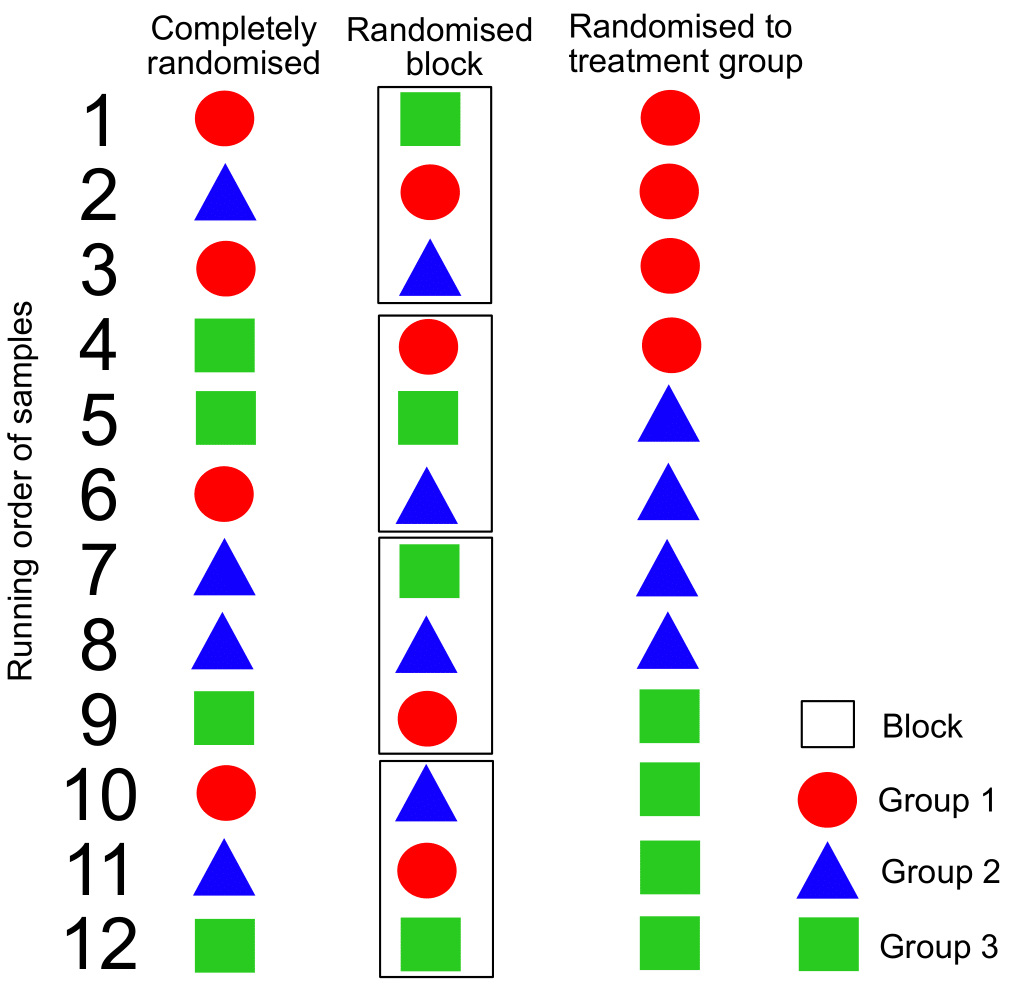
Sometimes several sources of variation are combined to define the block, so the block becomes an aggregate variable. Consider a scenario where we want to test various subjects with different treatments. Assume that we can divide our experimental units into \(r\) groups, also known asblocks, containing \(g\) experimental units each.Think for example of an agricultural experiment at \(r\) different locationshaving \(g\) different plots of land each. Hence, a block is given by a locationand an experimental unit by a plot of land. In the introductory example, a blockwas given by an individual subject.
Error
Therefore, one can test the block simply to confirm that the block factor is effective and explains variation that would otherwise be part of your experimental error. However, you generally cannot make any stronger conclusions from the test on a block factor, because you may not have randomly selected the blocks from any population, nor randomly assigned the levels. A special case is the so-calledLatin Square design where we have two blockfactors and one treatment factor having \(g\) levels each (yes, all of them!).Hence, this is a very restrictive assumption. In a Latin Square design, eachtreatment (Latin letters) appears exactly once in each row and once ineach column.
Example Problem on Randomized Complete Block Design
We can test for row and column effects, but our focus of interest in a Latin square design is on the treatments. Just as in RCBD, the row and column factors are included to reduce the error variation but are not typically of interest. And, depending on how we've conducted the experiment they often haven't been randomized in a way that allows us to make any reliable inference from those tests.
When should you use blocking?
The degrees of freedom for error grows very rapidly when you replicate Latin squares. But usually if you are using a Latin Square then you are probably not worried too much about this error. The error is more dependent on the specific conditions that exist for performing the experiment. For instance, if the protocol is complicated and training the operators so they can conduct all four becomes an issue of resources then this might be a reason why you would bring these operators to three different factories. It depends on the conditions under which the experiment is going to be conducted.
Randomization, Blocking, and Re-Randomization by Leihua Ye, PhD - Towards Data Science
Randomization, Blocking, and Re-Randomization by Leihua Ye, PhD.
Posted: Sun, 21 Nov 2021 08:00:00 GMT [source]
Irrigated barley–grass pea crop mixtures can revive soil microbial activities and alleviate salinity in desertic conditions ... - Nature.com
Irrigated barley–grass pea crop mixtures can revive soil microbial activities and alleviate salinity in desertic conditions ....
Posted: Mon, 14 Aug 2023 07:00:00 GMT [source]
Somecommon control variables are technical in nature, such as proteasebatches, freezer locations, and biobanks, but sample characteristicssuch as sex, age, and patient ancestry also commonly fall into thiscategory. As the complexity of the design increases, it is commonthat not all samples can be processed at the same time in the sameway at the same location. The sets of samples created by this processare referred to as batches, and this becomes yet another control variableto account for. Many times there are nuisance factors that are unknown and uncontrollable (sometimes called a “lurking” variable). We always randomize so that every experimental unit has an equal chance of being assigned to a given treatment. Randomization is our insurance against a systematic bias due to a nuisance factor.
Crossover Design Balanced for Carryover Effects
A nuisance factor is a factor that has some effect on the response, but is of no interest to the experimenter; however, the variability it transmits to the response needs to be minimized or explained. We will talk about treatment factors, which we are interested in, and blocking factors, which we are not interested in. We will try to account for these nuisance factors in our model and analysis.
ANOVA Summary Table
Often, variablesare continuous or categories are overlapping, such as age or diseasestate, respectively. In both cases categorization is commonplace,but can be problematic. Categories that span a large number of valuescan lead to relatively large differences between subjects within acategory, while the differences between subjects at the edges of neighboringcategories will be small. On the other hand, in a large enough studythe randomization should mitigate this problem.
The strategiesoutlined for the theoretical settings above caneasily be extended to more elaborate situations. However, as for allmethods, implementing block randomization can quickly become challengingin real-world situations. In this section, special considerationsare introduced for situations where the reality of the experimentposes challenges in experimental design. When the experiment consists of multiple batches,they will undergothe same experimental protocol at different points in time and/orspace. Every step of the protocol may then introduce variation thatis specific for each batch. If samples are moved across batches betweenprocessing steps, each processing step has its own specific sample-to-batchallocation.
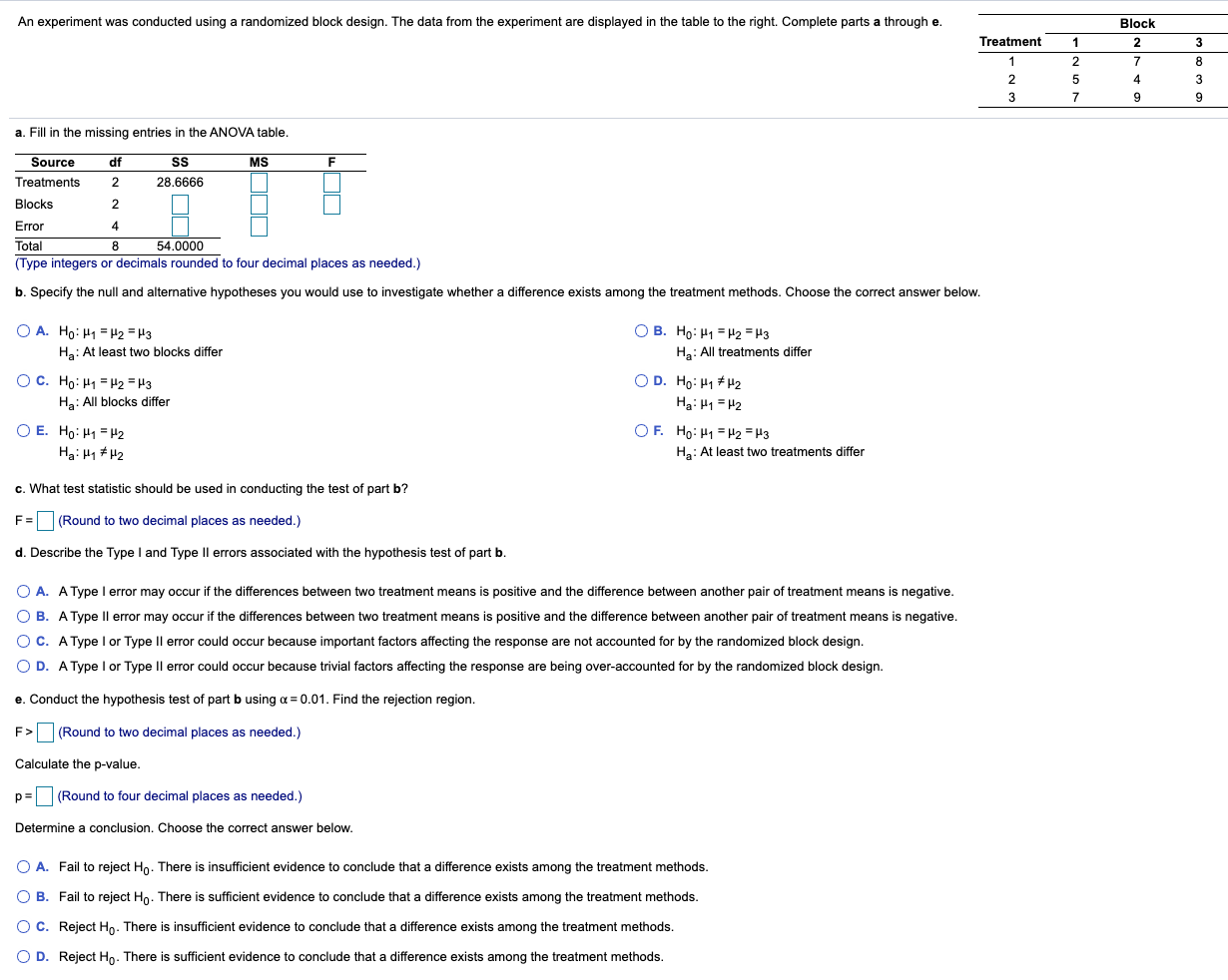
Book traversal links for 4.1 - Blocking Scenarios
Here we have used nested terms for both of the block factors representing the fact that the levels of these factors are not the same in each of the replicates. Both the treatments and blocks can be looked at as random effects rather than fixed effects, if the levels were selected at random from a population of possible treatments or blocks. We consider this case later, but it does not change the test for a treatment effect. The Analysis of Variance table shows three degrees of freedom for Tip three for Coupon, and the error degrees of freedom is nine. The ratio of mean squares of treatment over error gives us an F ratio that is equal to 14.44 which is highly significant since it is greater than the .001 percentile of the F distribution with three and nine degrees of freedom. Back to the hardness testing example, the experimenter may very well want to test the tips across specimens of various hardness levels.
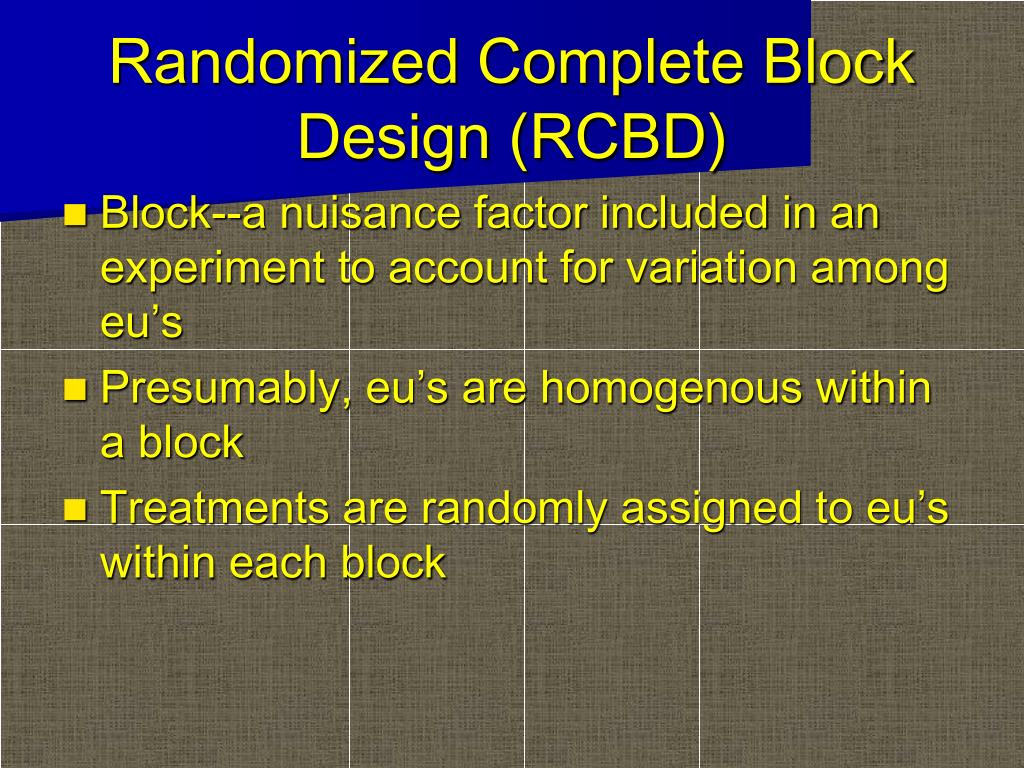
Basic residual plots indicate that normality, constant variance assumptions are satisfied. Therefore, there seems to be no obvious problems with randomization. These plots provide more information about the constant variance assumption, and can reveal possible outliers. The plot of residuals versus order sometimes indicates a problem with the independence assumption. In some disciplines, each block is called an experiment (because a copy of the entire experiment is in the block) but in statistics, we call the block to be a replicate. This is a matter of scientific jargon, the design and analysis of the study is an RCBD in both cases.
Use the viewlet below to walk through an initial analysis of the data (cow_diets.mwx | cow_diets.csv) for this experiment with cow diets. The following crossover design, is based on two orthogonal Latin squares. This situation can be represented as a set of 5, 2 × 2 Latin squares.
However, the presence of these factors (also known as nuisance factors) will introduce systematic variation in the study. For example, the crops produced in the northern vs the southern part will get exposed to different climate conditions. Controlling these nuisance factors by blocking will reduce the experimental error, thereby increasing the precision of the experiment and many other benefits. In the completely randomized design (CRD), the experiments can only control the random unknown and uncontrolled factors (also known as lucking nuisance factors). However, the RCBD is used to control/handle some systematic and known sources (nuisance factors) of variations if they exist.
No comments:
Post a Comment